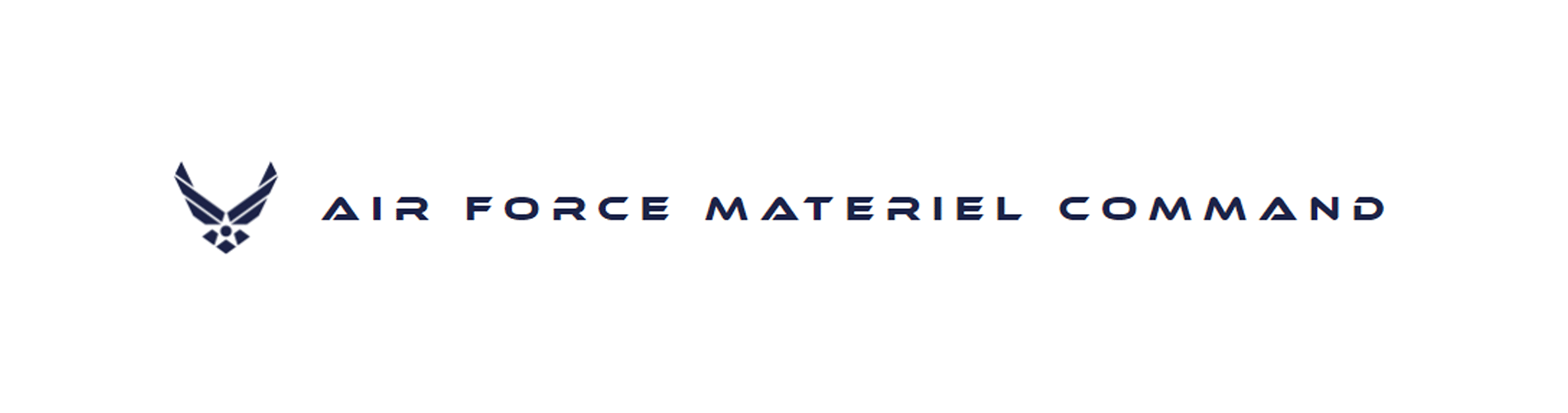
PARSERS: Privacy-preserving Analytics for Recognizing the Signs of an Elevated Risk for Suicide
Contract FA8222-21-C-0003, Air Force Materiel Command (Hill Air Force Base), 6/16/2021-11/21/2023
Technical Abstract
The critical nature of employee health and wellness is well documented in scientific and popular organizational literature. Recently, researchers have developed work wellness models, which lend themselves to better articulation of wellness within work environments. No matter the model, employee health and wellness factors have a clear impact on individual employee and organizational outcomes.
Organizations have a “duty of care” to their employees, and need to consider, monitor, and work to manage the health and wellness of employees. Active monitoring and management of wellness factors by leaders, managers, mentors, advocates, and other responsible personnel can have a clear impact on the overall wellness of employees. Unfortunately, employees are not always willing to communicate the status of their health and wellness, nor are managers always in the right place at the appropriate time to observe factors related to employee health and wellness. Data-enabled technology that can unobtrusively monitor and alert managers of wellness issues could be particularly valuable.
The Privacy-preserving Analytics for Recognizing the Signs of Elevated Risk Situations (PARSERS) approach seeks to form a bridge between health and wellness domain knowledge, USAF human resources (e.g., Wingman Advocates), and technology solutions that will form the foundation of a sustainable capability to support workplace health and wellness, from individual performance to severe clinical situations. PARSERS extends traditional machine learning methods by (1) deploying a privacy-preserving generative adversarial network (GAN) to create synthetic data capable of training accurate models that do not allow any individual to be reidentified; and (2) providing explainable models, where the key features that drive certain predictive outputs are able to be identified in terms of their importance. Coupled with intuitive analytic user interfaces for end users (e.g. Wingman Advocates, clinicians, and/or Wing Commanders) using mobile and desktop devices, this combination of privacy preservation and explainable predictive modeling has the potential to improve the situational awareness of individuals responsible for promoting workplace health and wellness.
In Phase II of this effort, we will conduct deeper, focused evaluations of the variables and dynamics driving workplace health and wellness, and understand how recognizing patterns in personnel management system data can lead to actionable insights. Potential results include workplace performance improvement and the ability to recognize early warning signs of severe clinical situations such as suicide risk. The overall goal is to empower USAF personnel to facilitate healthier workplace wellness through a recognition of the factors that facilitate social-emotional health improvement. Our approach will effectively allow for “communicating through data” with data-driven wellness conversations.
Anticipated Benefits and Potential Commercial Applications
The end result of the PARSERS Phase II effort will be a prototype application and architecture for performing privacy-preserving, explainable machine learning operations on sensitive data from USAF systems to predict factors related to workplace health and wellness. USAF end users will be able to gain insights from these complex modeling approaches about how to best serve the civilian and military operators supporting Air Force missions. PARSERS has wider applicability to address issues of occupational health in industry.
For a demonstration of or more information about PARSERS, please contact aptima_info@aptima.com.