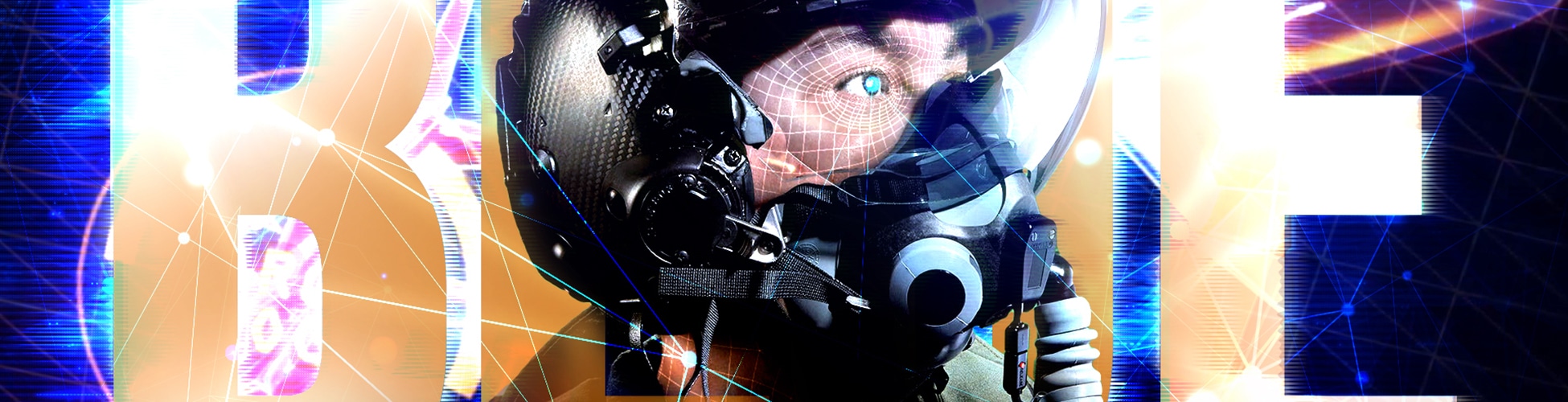
A Dream Team for Training? US Air Force Research Leads to New Ways for AI to Train Pilots
Despite AI imperfections, the ‘best of’ AI adversaries could improve Fighter Pilot training
Fighter pilots possess an extraordinary set of skills, developed in large part by engaging in simulated combat scenarios. In these ‘dogfights,’ trainees fly against human experts, where they learn to set up the enemy, manage their limited weapons and fuel, and avoid becoming a target themselves. But expert trainers are costly and in short supply. And alternatives, such as computer-generated forces, simply don’t provide such smart, robust competition.
This led the US Air Force to explore the potential of using AI to train pilots, that is, to fly against trainees as a human adversary might. After two years of experiments the results were spotty. Performance across the seven AI that the USAF tested varied widely. Any one AI agent might be an adept adversary in one flight scenario, and inept in another. And within a scenario, an AI agent could excel in some tactics and perform poorly in others.
The researchers from Aptima, which led the research for the Air Force, saw an opportunity, even a silver lining in those mixed results: exploit the variance in the AI so that the best suited AI could be matched to the scenario and trainee. To use a sports metaphor, a novice tennis player might best improve their game playing against one partner, and as they became more skilled, playing against a different partner. Similarly, one practice opponent might best develop their baseline game; the other, their serve-and-volley. The researchers in this study posited that with some innovative selection strategies, the best AI could be chosen from many to best fit the training task at hand.
These findings were selected for the Best Paper Award at The NATO Modelling and Simulation Group 2020 Symposium, a conference focused on training innovations. The implication of the team’s research was that the Air Force could exploit AI’s inconsistencies and variability to train pilots more effectively and economically.
The training problem – and why AI
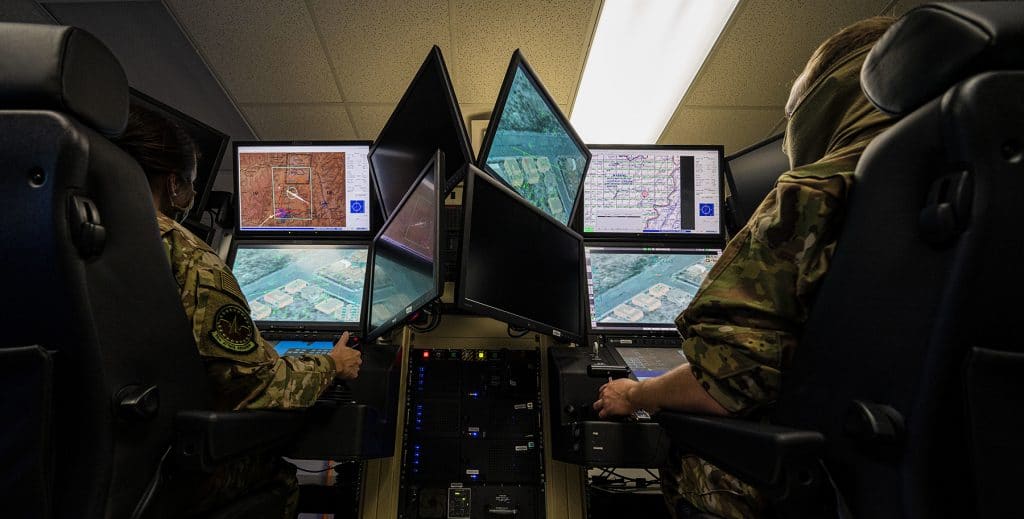
Photo courtesy www.af.mil (Released)
Some of the most effective training is for pilots to fly against human experts in simulators. These trainers respond smartly and adroitly as they put trainees through their paces in combat scenarios. But there simply aren’t enough expert trainers to go around for pilots to train frequently enough or be available on-demand.
Computer Generated Forces (CGFs) are far more accessible for training purposes. However, these adversaries, implemented in software, perform to a static script. CGFs are simplistic. As trainees become proficient, they can ‘game’ the CGFs, countering their more predictable moves. Novice trainees can err to the point that CGFs cannot respond. This limits their training value.
AI as Opponent?
AI has proven to be a worthy adversary. It has gone head-to-head and beaten the world’s best in some of the most complex games, including chess and the Chinese game of Go. AI participated unevenly but well in simulated aerial combat in DARPA AlphaDogfight.
To pursue AI for pilot training, the Air Force Research Laboratory (AFRL) created the AI research program, the “Not So Grand Challenge”. (The name is a nod to the program’s modest means, not its goal). If AI pilots could be developed to be adroit like humans, and less gameable than CGFs, it could present a breakthrough opportunity. Synthetic AI adversaries could make pilot training higher quality, more accessible, and lower cost.
The Challenge
AFRL sponsored eight US firms, led by Aptima, to develop AI pilots designed to fight smartly across a range of simulated battles. These ‘Red’ AI pilots were then flown on a testbed, conducting aviation tactics against Blue opponent in 70+ scenarios. Each scenario was a variation in setup, in how the planes relate in space, their weapon loads, available fuel, and other constraints that would affect their tactical decision-making. The testbed, developed by Aptima, was specially designed to generate the data to compute the AI’s tactical decisions, while capturing their behaviors in simulated flight. The Red AI pilots were scored on 11 dimensions, including their kill ratios (Blue kills vs Red losses), fratricide, the amount of time spent inside an opponent’s lethal weapon range, fuel and weapons management, targeting/sorting, and overall tactical intelligence.
The Findings: Not All AI are Equal
After two years of development and testing, the assessments showed a huge variance in the performance of the seven AI pilots. Each AI pilot excelled in some scenarios and performed poorly on others. Each AI also varied in its tactical skills. No one AI agent excelled in all scenarios, meaning, none was found to be the ideal training partner across all scenarios.
While AI has proven to be unbeatable in chess, it turns out its difficult to build such AI in aviation. And even if it could be, its instructional value would be questionable. An unbeatable AI pilot might simply overwhelm most trainees — and that would hinder training.
The Goldilocks Principle of Training
Training is often most effective when it meets the student’s skill. The adversary should be at a level that’s not too difficult, not too easy, but in a zone that’s just right. Imagine practicing against tennis great Rafael Nadal as he continually blasts aces past you. It is hard to discover any move that improves your score against Nadal. It’s hard to learn for all the losing. Trainees don’t learn well if they’re defeated each and every time. Conversely, if training is too easy, those skills may not advance in training; in operations poor tactical skills can get pilots killed.
This is where the researchers recognized the value of the AI variance, where their range in performance could be exploited for training value. With the seven AI scored and characterized by what they did well, and not so well, the researchers reasoned the AI could be selected or matched to the scenario and trainee. Cataloging the AI in a library of sorts, the most competent AI, and ideally most instructionally impactful one, could be chosen as training adversary. To the tennis example, it would be like selecting the training partner to best develop that aspect of your game as your skills advanced.
The researchers posited that an automated librarian could accomplish this, but how would it work?
Choosing Smartly between AI for the Training Need
The research team devised several schemes for how to automate this AI selection. These approaches ranged from applying simple rule sets to select the agent to the scenario; to using the recommendations of training experts; to applying complex models to select both agents and scenarios to instruct trainees as they proceed. These approaches, which vary in cost and instructional effectiveness, are ordered below, least to most.
- Selecting the AI by rule: The automated librarian would select the AI that performs best on the training scenario, or a discrete part of the scenario that the trainee is about to execute. For example, it might choose the aggressor AI that kills Blue pilots most frequently when Blue turns away and its fuel is low. This would allow the librarian to swap agents within scenarios to ensure that the sharpest AI is engaged in each vignette.
- Selecting by expert judgment: This automated librarian would make the selection based on the predictions of training experts concerning the likelihood each AI would advance specific trainee skills. For example, a particular Red AI might be expected to stretch and grow the skills of novice pilots, but not be effective for experienced ones. During training, the librarian would select the AI projected to have the greatest instructional impact for that trainee and scenario.
- Selecting by probabilistic model: This librarian uses a formal computational model to select both the best AI agent and scenario for a trainee. This model leverages training data in real time. As performance measurements roll in, it selects the next scenario and agent that will advance the trainee to expertise most quickly and surely. These ‘adaptive learning models,’ which have been demonstrated in military
In sum, although the AI developed for the Challenge were designed to win, not specifically to train, Aptima’s researchers found a way to exploit the variance across the AI for the goal of training fighter pilots.
The ability to choose the best AI for the training task would like choosing the best human adversary to train a pilot, or more generally, choosing the best instructor for the training mission and trainee. Currently, the Air Force does not have the ability to do either. If AI is remotely as adroit as a training adversary, then the ability to select the best AI will be a significant new capability as the Air Force looks to improve access to quality training.
To download a free PDF copy of the paper courtesy of the NATO Science and Technology Organization (STO) click on the following link: Assessing & Selecting AI Pilots for Tactical and Training Skill.
Full citation: Freeman, J., Watz, E., and Bennett, W. (2020). Assessing & Selecting AI Pilots for Tactical and Training Skill. Proceedings of the 2020 NATO Modelling & Simulation Group (NMSG) Symposium. Virtual event.
The material reported here was assigned a clearance of CLEARED for domestic and international audiences on 28 Aug 2020 in Case Number: 88ABW-2020-2719.